PREDICTIVE ANALYTICS FOR PATIENT OUTCOMES
Course 5406
4 Hours
$399
Predictive analytics is reshaping patient care. This course equips healthcare professionals and data scientists with the skills to build and apply predictive models, improving outcomes through data-driven insights.
Target Audience
- Clinicians and healthcare administrators interested in data-driven care improvements
- Data scientists and analysts working in healthcare settings
- IT professionals supporting healthcare analytics initiatives
- Healthcare executives exploring advanced analytics solutions
Course Format:
- Live Virtual Lectures: Delivered by experts in healthcare analytics.
- Interactive Activities: Hands-on exercises, case studies, and scenario planning.
- Resources Provided: Datasets, predictive model templates, and evaluation guides.
Payment Information: We accept credit card payments.
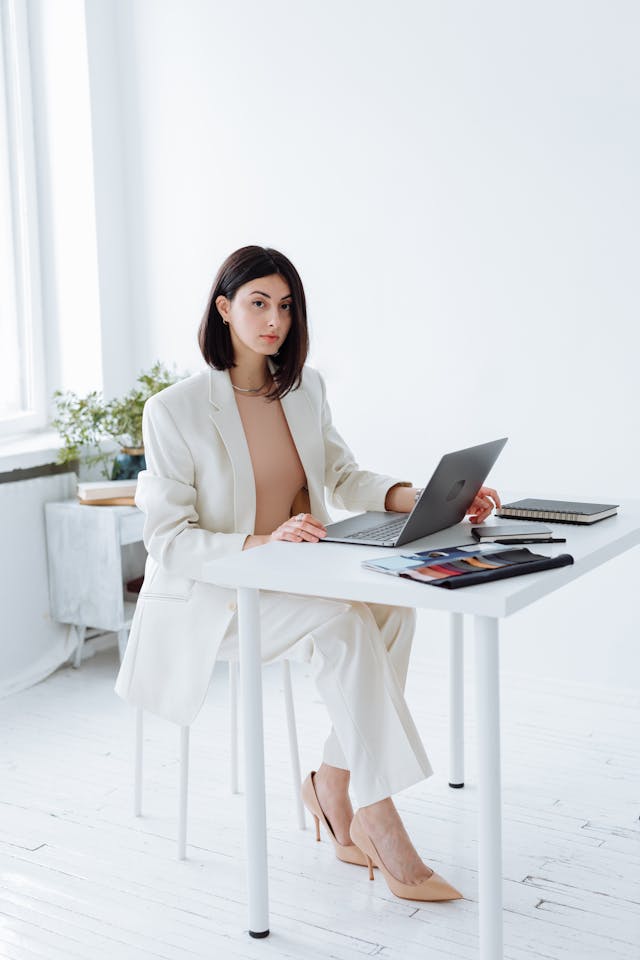
Course Objectives
- Understand the fundamentals of predictive analytics in the healthcare context.
- Learn to build and evaluate predictive models tailored to patient care.
- Explore real-world applications for forecasting patient risks and outcomes.
- Address challenges in implementing predictive analytics while maintaining ethical standards.
Course Structure:
Introduction to Predictive Analytics in Healthcare
- Overview of predictive analytics: key concepts and definitions.
- Importance of predictive analytics in improving patient outcomes.
- Common use cases: readmission prediction, risk stratification, and treatment optimization.
Building Predictive Models for Patient Outcomes
- Selecting appropriate algorithms: regression, decision trees, and ensemble methods.
- Preprocessing patient data for predictive modeling: handling missing values and outliers.
- Training, testing, and validating predictive models for accuracy.
Evaluating and Interpreting Predictive Models
- Metrics for model evaluation: sensitivity, specificity, AUC-ROC, and more.
- Interpreting model results to draw actionable insights.
- Communicating predictions effectively to clinical teams and stakeholders.
Real-World Applications and Challenges
- Case studies: using predictive analytics for readmission prevention, sepsis detection, and chronic disease management.
- Challenges in implementing predictive analytics: data privacy, integration, and clinician adoption.
- Strategies for integrating predictive models into healthcare workflows.
Ethical Considerations and Future Trends
- Addressing ethical concerns: bias, transparency, and patient consent.
- Regulatory considerations: HIPAA, GDPR, and FDA guidelines for predictive tools.
- Emerging trends: real-time analytics, personalized medicine, and AI-driven models.
Group Rates Available!
Bring your team and save – perfect for healthcare organizations looking to train multiple leaders.
Discounts available for organizations enrolling 5+ participants.
Class Schedule
Rates below are for each participant.
Frequently Asked Questions
What will I learn in this course?
You’ll learn to build predictive models, evaluate patient risks, and explore real-world applications for improving patient outcomes using data-driven techniques.
Who should take this course?
This course is ideal for clinicians, administrators, data scientists, and IT professionals involved in healthcare analytics or patient care strategies.
Do I need prior experience with predictive analytics?
No prior experience is required. The course introduces concepts and tools in an accessible way, with hands-on practice included.
Will I build a predictive model during the course?
Yes, participants will create a simple predictive model using a provided patient dataset and learn to evaluate its performance.
What tools will I use during the course?
Participants will work with Python libraries (e.g., scikit-learn) or Excel-based tools, depending on their experience level and organizational resources.
How does the course address real-world challenges?
The course includes case studies and scenario-based exercises to tackle challenges like data privacy, model integration, and clinician adoption.
Does the course cover ethical concerns?
Absolutely. A dedicated module focuses on ethical considerations, including bias, transparency, and compliance with healthcare regulations.
What kind of datasets will I work with?
You’ll work with anonymized patient datasets to ensure privacy while learning practical modeling techniques.